Look before you leap
How to decide when GenAI is the right solution — and when it’s not
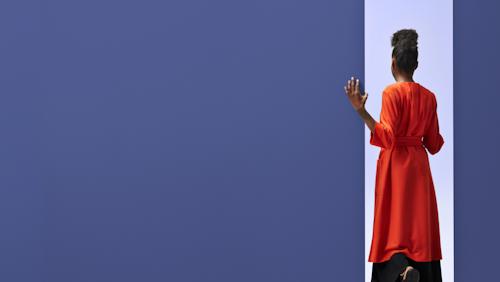
Every day, another business proudly announces its embrace of AI, promising revolutionary improvements in productivity and efficiency. But beneath the hype lies a hard truth: AI isn’t a magic solution that can solve every problem.
And the risks of wasted resources and derailed goals are very, very real. Gartner estimates that 30% of GenAI projects will be abandoned after proof of concept by the end of 2025, due to poor data quality, inadequate risk controls, escalating costs or unclear business value. “There’s been an expectation that AI is a silver bullet, and it’s going to quickly and easily solve all your problems,” says Austin Humes, Director of Technology and Connected Experiences at Valtech. “And that’s not the case whatsoever.”
Implementing AI effectively requires thought and intention on the front end. Before committing to any new AI solution, you must carefully evaluate your needs, your available resources and potential alternatives.
Focus on real business problems
Before you get too excited about a tool, identify the specific problems you hope to solve with AI and what outcomes you want to achieve.
You need to generate ROI targets for the outcomes of a project before committing new or additional resource,
- says Cameron Turner, Vice President of Data Science at Valtech
For UK-based retailer Matalan, that pain point was an overloaded copywriting team that struggled to keep up with descriptions for their vast product range. They decided to test whether GenAI could alleviate some of that workload while delivering better search results to its customers. The payoff was quick: In three weeks, they stood up a GenAI and large language model (LLM) solution with Google Cloud Platform's Gemini and PaLM 2 on Vertex AI. The new AI-assisted process lets writers produce 100 descriptions every 30 minutes. Before, 100 descriptions was a day's work for the copywriting team.
However, as you solve one problem, don’t forget to consider how that solution may impact other areas of the business. Many organizations stitch together disparate AI solutions, leading to fragmentation that undermines AI’s overall potential. "The moment you cobble together multiple point solutions without a clear strategy or cohesive architecture, the benefits of those individual tools are lost. Any efficiency or productivity gains evaporate,” says John Cunningham, Chief Technology Officer at Valtech.
Be realistic about your AI-readiness
GenAI costs go beyond setup, with ongoing expenses for software, hardware and support — and you may not always see an immediate return. Or a return at all, if you’re not thoughtful about the project. “The hype says 3000% return. That's highly unlikely. There's lots to consider that could impact results in the long run and validating through the lifetime of a project is critical,” says Morgan Kainth, VP Strategy – Europe, Valtech.
Your odds of success go up significantly if you already have robust data storage, processing power and access to high-speed networking. Even with sufficient hardware, disorganized data remains a challenge. “They have the data, but we’re finding that it’s not necessarily organized in the right ways,” says Lindsay Ratcliffe, MD UK and SVP Product, Innovation and Data Services Europe at Valtech.
A skilled workforce — data scientists, AI engineers and developers — is also critical. If internal talent is lacking, hiring or training will be needed. Most leaders (82%) in Microsoft’s 2023 Work Trends Index agree that new skills are essential for working with AI.
"AI needs continuous improvement, monitoring and maintenance. The more it is properly leveraged in a business, the more human labor you will need,” says Lisa Rogers, Ph.D., Director of Data at Gumband, a software platform that helps manage and measure interactive experiences in a variety of settings, including museums, retail stores and stadiums. “Skills and expertise that are most critical for implementing and maintaining AI solutions include mathematical modeling, neurological modeling, data analysis skills and LLM building knowledge.
Cunningham agrees that skills building is key: “Do internal training around prompt engineering across everything from image generation to copy generation to show people the differences between the different types of prompt approaches.”
Don’t forget about non-AI alternatives
If your resources are limited, alternative solutions may be more practical. Basic automation can handle repetitive tasks, while data analytics helps businesses extract insights to improve decision-making and efficiency. These can provide significant benefits without the high initial investment and ongoing costs of AI.
"We spend time tempering the euphoria of executives towards the technology and instead refocusing together on the business objective. And only then backing into the technology needed,” Turner says. “Because sometimes GenAI isn’t warranted. Sometimes the certainty of fact-based analytics outweighs the benefits of probability-based AI predictions.”
Not every challenge requires an AI solution. “A lot of smaller, static problems can be solved with simpler automation or data analytics that are more cost-effective and faster to implement,” says Rumi Morales, partner and board member at Outlier Ventures. “AI is better suited for large-scale, dynamic issues that require continuous adaptation.”
For example, Danish rental service Findbolig needed to modernize an outdated legacy system. Rather than opting for a complex AI solution, they focused on an API-based approach to streamline processes and reduce operational strain. This solution freed up internal resources, allowing the team to concentrate on more strategic initiatives while still improving efficiency. By selecting a practical, non-AI alternative, Findbolig managed to update their systems without overextending resources or investing in unnecessary technology.
Stick the landing
AI has the potential to revolutionize the way businesses operate, but that doesn’t mean it’s always the perfect solution for everyone. The real key to success is taking the time to reflect on what your business needs. Does AI fit with your goals? Do you have the resources to make it work?
Sometimes, simpler options like automation or data analytics can deliver big results without massive investment. It’s all about finding the right tool for the job. So, before diving headfirst into AI, ask yourself: Is this really the smartest move for your business right now?